Overview:
Sqoop is a tool used for data transfer between RDBMS (like MySQL, Oracle SQL etc.) and Hadoop (Hive, HDFS, and HBASE etc.)It is used to import data from RDBMS to Hadoop and export data from Hadoop to RDBMS.
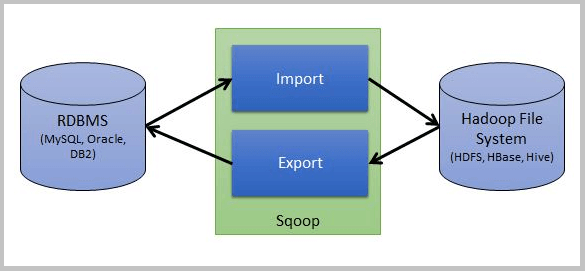
Sqoop Architecture:
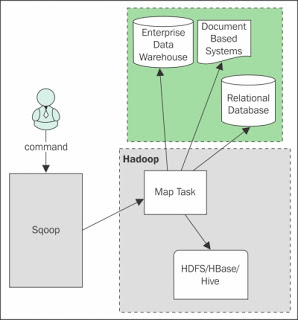
When Sqoop starts functioning, only mapper job will run and reducer is not required. Here only Map phase will run and reduce is not required because the complete import and export process doesn’t require any aggregation and so there is no need of reducers in Sqoop.
SQOOP IMPORT
Sqoop import tool is used to import the tables from a relational database like MySQL, Oracle SQL etc.to the Hadoop system like Sqoop import to HDFS or Hbase etc.
Each row of an RDBMS table is treated as the records of the file. It will store text data in text file and binary data in sequence file or Avro file.
For example, I have one table as below-
The table name is “emp” in the database “userdb” in MySQL database server
Now we will import this table emp data into HDFS. Let’s see how easy it is-
Syntax
-------------
$ sqoop import --connect jdbc:mysql://<mysql-server-hostname>/<database-name> --username <username-of-mysql-db> --table <table-name> -n <number-of-mappers>
-------------
Command
--------
$ sqoop import –connect jdbc:mysql://localhost/userdb –username root –table emp m 1
-----
Once you will run the above commands, you will find it running and using some mappers. Once done, you can also see the output using below commands-
------
$HADOOP_HOME/bin/hadoop fs -cat /emp/part-m-*
------
You will find the output file in HDFS something like below. It will be a comma (,) separated.
101, John, 5000, IT, Developer
102, Chris, 6000, Sales, Manager
103, Jamie, 7000, Support, Director
Using the above command, the file will be saved in a default location in HDFS.
Import Table data in a particular location
But what if we want to save the file in a particular location (let’s say rdbmsdata) in HDFS?
You can specify the location (target directory) in above command. For this, we use the below command-
–target-dir /rdbmsdata
So the complete command will be something like below-
$ sqoop import --connect jdbc:mysql://localhost/userdb --username root --table emp --m 1 --target-dir /rdbmsdata
Verify the result using the below command-
-------
$HADOOP_HOME/bin/hadoop fs -cat /rdbmsdata/part-m-*
-------
Incremental Import
This section will be highly helpful to you if you are using on real time data. For example, you are working on some employee data of a company and everyday new hiring is getting done and so new employee will get added as well.
So in such situation, you will only want to import only the newly updated records and in such case incremental import will help you a lot.
To get this done, you need mainly three things-
Incremental- Mode like appended
check-column – based on which column you want to decide the incremental value
last value – What was the last value you imported so that it will take next value after that
Now considering a new employee has been added in the emp table with the record as below-
------
104, Henning, 4000, IT, Developer
------
And we want to take ID column as the check column and so the last value was 103 and the new is 104.
The complete query to import the newly added rows in the table emp.
Let’s see the query-
------
sqoop import --connect jdbc:mysql://localhost/userdb --username root --table emp
--m 1 --incremental append --check-column id --last-value 103
------
Again you can check the output using the below command-
$HADOOP_HOME/bin/hadoop fs -cat /emp/part-m-*
As you have seen so far, the result will be comma separated by default while importing the data from the relational database to Hadoop.
In all the above examples, I have shown you how to import table in HDFS through Sqoop.
How to Import Table Data in HBase with Sqoop?
Like we imported table in HDFS through Sqoop, in a similar way we can do for Hbase as well but there is some twist.
Sqoop doesn’t now permit you to import a relational table directly into an HBase table having multiple column families. To work around this limitation, you can create the HBase table first and then execute three Sqoop import operations to finish the task.
Let’s start by creating an Hbase table first-
Create ‘tablename’, ‘columnfamily’
For example: create ‘empdetails’, ‘personal data’, ‘professional data’
Once you have created a table in HBase, you are all set to write the command to import the RDBMS table into Hbase through Sqoop. Below is the code-
-----
sqoop import --connect jdbc:mysql://localhost/serviceorderdb --username root -P --table emp --columns “designation,name” --hbase-table empdetails --column-family personal details --hbase-row-key ID -m 1
-------
Once executed, you can scan the HBase table to check the output.
------
Scan empdetails;
-----
In the next post I'll write the procedure to export data from Hadoop to RDBMS.